In the world of predictive analytics, data is the main element. However, not all data is valid or can be useful to us. The data must be relevant, accurate and clean.
It has currently become a powerful tool in data analytics, offering organizations the ability to forecast trends, optimize strategies and anticipate results.
Data analysis may seem like a complicated task, but it doesn’t have to be. Today we bring 5 predictive steps that can simplify your data analysis.
What is predictive analytics?
Predictive analytics is a branch of advanced analytics that uses historical data, statistical modeling, data mining techniques, and machine learning to make predictions about future outcomes.
In the context of data analytics in factories, it can involve predicting production trends, identifying potential maintenance issues before they occur, optimizing the factory…
But how can predictive analysis be simplified and have great value?
1. Define your objectives
The first step in any data analysis is to clearly define the problem you are trying to solve. At this time it is important to establish clear objectives for your analysis.
Set clear goals for data analysis
Healthy employees tend to be more productive and have fewer absences, resulting in greater continuity in the production chain.
Define what you want to achieve with your efforts. Through this step, you will be able to generate focus on what really matters, select appropriate tools, communicate results effectively, and make better data-driven decisions.
It is important to always keep in mind that the objectives set in data analysis must be specific, measurable, achievable, relevant and with a specific deadline, so that they are truly useful.
Having a solid approach will offer the possibility of guiding your data analysis in the right direction.
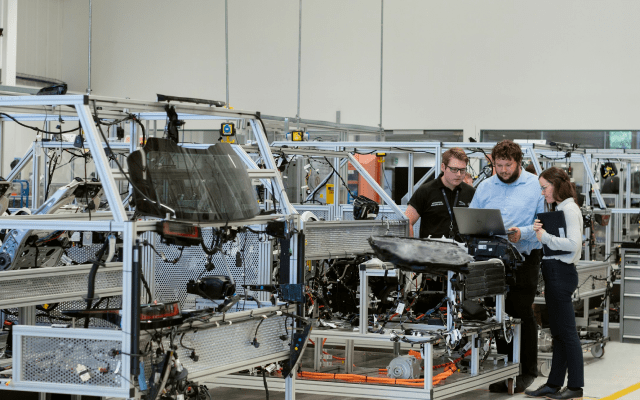
2. Data collection and cleaning
Once we have the problem well defined, the next step is to collect the data needed to solve it. This may involve collecting existing data, conducting surveys or experiments.
Obtaining relevant data
It is important to collect data that is relevant to the problem you are trying to solve. You must ensure that you collect data that is relevant to the proposed objectives and that is of high quality.
Preprocessing and data cleaning techniques
Before you can extract information from the data, your data must be cleaned and processed to extract relevant information. In this step, all errors, outliers and inconsistencies must be removed from the data.
Having dirty or incorrect data can lead to erroneous conclusions and inaccurate predictions.
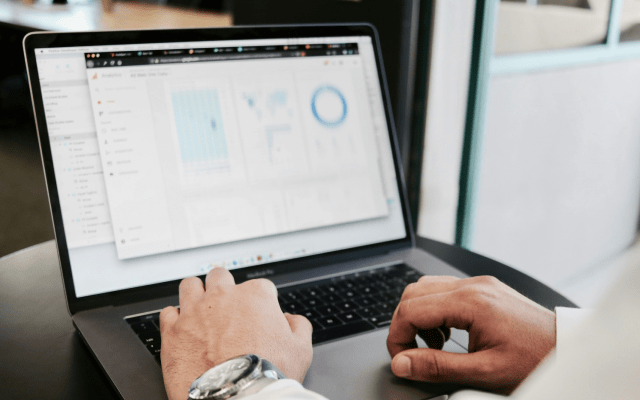
3. Exploratory data analysis
By exploring and summarizing the characteristics of a data set, you can better understand its structure and patterns. Understanding the distribution of data is essential since:
- Helps identify anomalies and patterns
By visualizing the distribution of data, we can identify general patterns and trends in the data. It can help us detect outliers, input errors, or unexpected behavior. - Select appropriate modeling techniques
The distribution of the data can influence the choice of appropriate modeling techniques. Some machine learning algorithms assume that data follows a normal distribution, while others are more robust to deviations from this norm. - Interpret model results
By understanding the distribution of the data, we can better interpret the results of predictive models.
4. Model selection and training
Once a model has been selected, the next step is to train it with your own data. During this process, the model focuses on “learning” from the data and adjusting its parameters to minimize prediction error.
Precision, complexity, and interpretability must be considered to choose the model that fits your data.
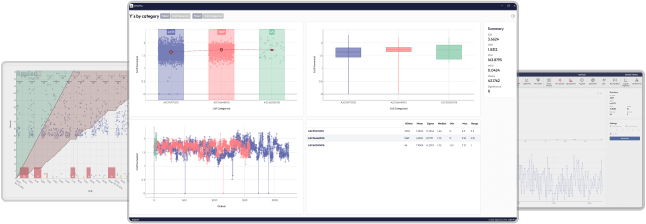
5. Evaluation and implementation
Finally, the performance of your model must be assessed. This may involve using metrics such as precision, recall, and value, as well as performing cross-validation to ensure that your model generalizes well to new data.
Conclusion
In today’s data-driven world, organizations of all sizes are harnessing the power of how data is used to make more informed decisions and achieve better results.
Predictive analytics can be a complex process, especially for those without experience in data science or statistical analysis.
Through these five steps, you will be able to clearly define your objectives, prepare your data, choose the right predictive model, train and evaluate your model, and deploy it.
Share this post