In the era of Industry 4.0, rapid implementation of analytical models can transform manufacturing processes, improving efficiency and reducing costs. But what steps do we need to take for rapid and successful implementation?
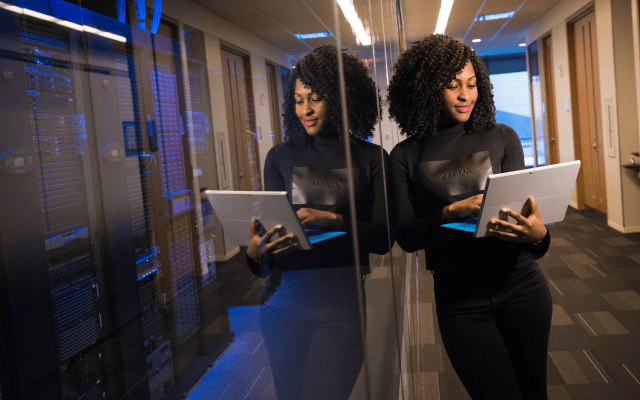
Actions to successfully implement an analytical model
- Define the Problem
Start by identifying a specific problem in manufacturing that needs optimisation, such as reducing downtime, improving product quality or resource efficiency. - Data Collection
Collect real-time data through sensors and IoT devices embedded in your manufacturing equipment. It is crucial that the data is of high quality and relevant to the problem identified.Use platforms such as RTM Pro to centralise and manage the collected data, ensuring it is available for further analysis. - Select Analytical Tools
Use RTM Pro to manage the data collected. This tool allows for quick and easy configurations, offering the flexibility to adjust to your specific needs. - Prepare the Data
If your company does not have a specialised department or trained staff, take advantage of AppliediT’s data engineering services to extract data from various sources and implement ETL processes to cleanse and transform the collected data. This process includes normalising data, handling missing values and removing irrelevant data, thus ensuring the quality and relevance of the data for modelling. - Develop the Model
Select an appropriate Machine Learning algorithm for your problem (regression, classification, clustering, etc.). There are a variety of algorithms that can be tailored to the specific requirements of your manufacturing operation. - Train and Validate
Train the model using historical manufacturing data. Validate the model with a test data set to assess its accuracy and performance. Adjust model parameters as necessary to optimise model performance. - Implement and Monitor
Deploys the model in the production environment. Use RTM Pro’s monitoring capabilities to monitor model performance in real time and make continuous adjustments based on new data. - Scalability and Maintainability
Ensure the model is scalable to handle increasing volumes of data. Automate model updates and adjustments to adapt to changes in data and processes, ensuring relevance and accuracy over time.
Key Benefits
- Process Optimization: Reduced downtime and improved operational efficiency.
- Informed Decision Making: Based on accurate, real-time data analysis.
- Cost Reduction: Through the identification and elimination of inefficiencies in manufacturing processes.
Conclusion
Implementing an analytical model quickly is not only possible, it is essential to stay competitive in the modern manufacturing industry.
With the right steps and tools, such as RTM Pro and our data engineering services, you can transform your data into actionable decisions in minutes.
Share this post