As a business owner or manager, you are always looking for ways to improve your products and services. One way to do this is by using data analytics to improve product quality. Data analytics is the process of analyzing and interpreting data to gain insights and make informed decisions. By using data analytics, you can identify trends, patterns, and areas for improvement in your products and services. In this article, I will discuss the benefits of using data analytics for product quality improvement, different types of data analytics used for product quality improvement, steps to implement data analytics for product quality improvement, common mistakes to avoid when using data analytics for product quality improvement, case studies of successful implementation of data analytics for product quality improvement, tools and technologies for data analytics for product quality improvement, key performance indicators (KPIs) to track for product quality improvement, and training and development for data analytics for product quality improvement.
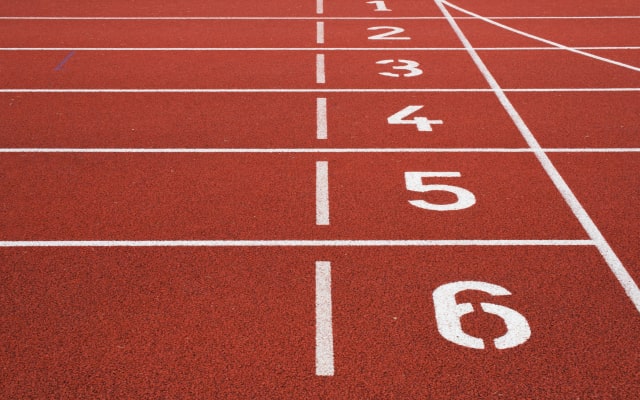
Benefits of Using Data Analytics for Product Quality
Using data analytics for product quality improvement has several benefits. Firstly, it helps you to identify areas for improvement in your products and services. By analyzing customer feedback, product reviews, and other data sources, you can identify patterns and trends in customer complaints and issues. This can help you to make targeted improvements to your products and services, which can increase customer satisfaction and loyalty.
Secondly, data analytics can help you to reduce costs associated with poor product quality. By identifying issues early on, you can take corrective action before the problem becomes widespread and costly. This can include identifying defective products before they leave the factory, or identifying areas where improvements can be made to reduce waste and inefficiency in the production process.
Thirdly, data analytics can help you to stay competitive in your industry. By using data to make informed decisions, you can adapt to changing market conditions and customer preferences. This can help you to stay ahead of your competitors and maintain a strong market position.
Different Types of Data Analytics Used for Product Quality Improvement
There are several different types of data analytics used for product quality improvement. The first type is descriptive analytics, which involves analyzing data to understand what has happened in the past. This can include analyzing customer complaints, product reviews, and other data sources to identify patterns and trends.
The second type is diagnostic analytics, which involves analyzing data to understand why something happened. This can include conducting root cause analysis to identify the underlying causes of product defects or customer complaints.
The third type is predictive analytics, which involves using data to predict what is likely to happen in the future. This can include using machine learning algorithms to predict which products are likely to fail or which customers are likely to churn.
The fourth type is prescriptive analytics, which involves using data to recommend the best course of action. This can include using optimization algorithms to identify the most efficient production processes or recommending specific improvements to product design based on customer feedback.
Steps to Implement Data Analytics for Product Quality Improvement
Implementing data analytics for product quality improvement can be a complex process, but there are several key steps that can help to ensure success. The first step is to identify the data sources that will be used for analysis. This can include customer feedback, product reviews, manufacturing data, and other sources.
The second step is to clean and prepare the data for analysis. This can involve removing duplicates, filling in missing data, and standardizing data formats to ensure consistency.
The third step is to conduct the analysis using the appropriate data analytics techniques. This can include descriptive, diagnostic, predictive, and prescriptive analytics, as discussed earlier.
The fourth step is to interpret the results of the analysis and make informed decisions based on the insights gained. This can include identifying areas for improvement in product design, manufacturing processes, or customer service.
The fifth step is to implement changes based on the insights gained from the analysis. This can involve making targeted improvements to product design, manufacturing processes, or customer service.
The sixth and final step is to monitor and evaluate the effectiveness of the changes made. This can include tracking key performance indicators (KPIs) to ensure that improvements are being made and that product quality is improving over time.
Common Mistakes to Avoid When Using Data Analytics for Product Quality Improvement
While data analytics can be a powerful tool for product quality improvement, there are several common mistakes that can undermine its effectiveness. The first mistake is failing to identify the right data sources for analysis. Without the right data, it is impossible to gain meaningful insights into product quality.
The second mistake is failing to clean and prepare the data properly. Without clean and consistent data, it is difficult to conduct accurate analysis and make informed decisions.
The third mistake is failing to use the appropriate data analytics techniques for the problem at hand. Using the wrong techniques can lead to inaccurate or incomplete insights.
The fourth mistake is failing to interpret the results of the analysis properly. Without a clear understanding of the insights gained, it is difficult to make informed decisions about how to improve product quality.
The fifth mistake is failing to implement changes based on the insights gained from the analysis. Without action, data analytics is just a theoretical exercise.
The sixth and final mistake is failing to monitor and evaluate the effectiveness of the changes made. Without tracking KPIs, it is difficult to know whether improvements are being made and whether product quality is improving over time.
Case Studies of Successful Implementation of Data Analytics for Product Quality Improvement
There are many examples of successful implementation of data analytics for product quality improvement. One example is a manufacturer of medical devices that used data analytics to improve the quality of its products. By analyzing data from customer complaints, manufacturing processes, and other sources, the company was able to identify areas for improvement in product design and manufacturing processes. As a result, the company was able to reduce the number of customer complaints and improve customer satisfaction.
Another example is a software company that used data analytics to improve the quality of its products. By analyzing data from customer feedback, product reviews, and other sources, the company was able to identify areas for improvement in product design and customer service. As a result, the company was able to increase customer satisfaction and loyalty.
Tools and Technologies for Data Analytics for Product Quality Improvement
There are many tools and technologies available for data analytics for product quality improvement. These include data visualization tools, statistical analysis software, machine learning algorithms, and optimization algorithms. Some popular tools and technologies include Tableau, SPSS, Python, and R.
Key Performance Indicators (KPIs) to Track for Product Quality Improvement
Tracking KPIs is essential for measuring the effectiveness of data analytics for product quality improvement. Some important KPIs to track include customer satisfaction, product defect rates, customer complaints, and production efficiency. By tracking these KPIs over time, you can monitor the effectiveness of your product quality improvement efforts and make adjustments as needed.
Training and Development for Data Analytics for Product Quality Improvement
Training and development are essential for ensuring that your team has the skills and knowledge necessary to use data analytics effectively for product quality improvement. This can include training in data analysis techniques, statistical analysis, and machine learning algorithms. It can also include ongoing professional development to ensure that your team stays up-to-date with the latest trends and technologies in data analytics.
Conclusion: Future of Data Analytics for Product Quality Improvement
Data analytics is an essential tool for improving product quality in today’s competitive business environment. By using data analytics to identify areas for improvement in product design, manufacturing processes, and customer service, you can improve customer satisfaction, reduce costs, and stay ahead of your competitors. To ensure success, it is important to avoid common mistakes, track KPIs, and invest in training and development for your team. The future of data analytics for product quality improvement is bright, and by embracing this technology, you can take your business to the next level.
If you are interested in implementing data analytics for product quality improvement in your business, contact us today to learn more about our services and how we can help you achieve your goals.
Share this post