Data analytics is a powerful tool that can help manufacturing companies improve their performance, efficiency, and profitability. However, for data analytics to be effective, it is essential that the data used is of high quality. Because low-quality data can lead to biased or inaccurate results, which can lead to erroneous decisions.
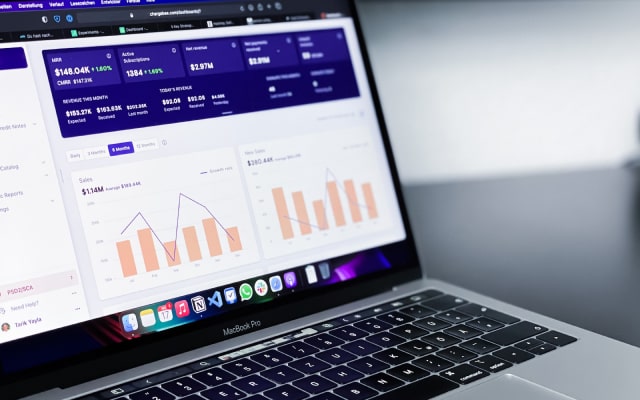
Data quality refers to the accuracy, consistency, and completeness of data. In the context of industrial manufacturing processes, data quality is especially important. The data used for data analytics in manufacturing is often complex and comes from a variety of sources, such as sensors, control systems, and production records.
Some of the most common data quality problems that can arise in industrial manufacturing processes include:
- Incomplete data: Data can be incomplete if not all relevant measurements are collected. This can make it difficult to identify trends and patterns.
- Inaccurate data: Data can be inaccurate if human errors are introduced or if faulty equipment is used. This can lead to incorrect results.
- Inconsistent data: Data can be inconsistent if it is collected from different sources or if different formats are used. This can make it difficult to combine data for analysis.
To ensure data quality in industrial manufacturing processes, it is important to implement the following measures:
- Define data requirements: Before collecting data, it is important to define data requirements. This will help ensure that the data collected is accurate, consistent, and complete.
- Implement robust data collection processes: Data collection processes must be robust to ensure that data is collected correctly. This includes using high-quality equipment and procedures.
- Perform data quality checks: Data quality checks should be performed regularly to identify and correct data quality problems.
By taking steps to ensure data quality, manufacturing companies can improve the accuracy and reliability of their analyses. This will help them make more informed decisions that can lead to improvements in performance, efficiency, and profitability.
Some benefits of data analytics with high-quality data in industrial manufacturing processes include:
- Improved efficiency: Data analytics can help manufacturing companies identify opportunities to improve process efficiency. For example, data analytics can help companies optimize resource utilization, reduce waste, and improve productivity.
- Reduced costs: Data analytics can help manufacturing companies identify opportunities to reduce costs. For example, data analytics can help companies improve product quality, reduce inventory, and improve customer satisfaction.
- Improved quality: Data analytics can help manufacturing companies improve the quality of products and services. For example, data analytics can help companies identify quality problems, improve manufacturing processes, and reduce defects.
- Improved decision-making: Data analytics can help manufacturing companies make more informed decisions. For example, data analytics can help companies predict demand, identify new markets, and develop new products and services.
In conclusion, data quality is essential for data analytics in industrial manufacturing processes. By taking steps to ensure data quality, manufacturing companies can improve the accuracy and reliability of their analyses. This will help them make more informed decisions that can lead to improvements in performance, efficiency, and profitability.
Share this post